The pursuit of carbon-free energy has largely leaned on intermittent sources of energy — like wind and solar; and sources that require a great deal of initial investment — like hydroelectric (which requires elevated bodies of water and dams) and nuclear (which require you to set up a reactor).
The theoretical beauty of geothermal power is that, if you dig deep enough, virtually everywhere on planet earth is hot enough to melt rock (thanks to the nuclear reactions that heat up the inside of the earth). But, until recently, geothermal has been limited to regions of Earth where well-formed geologic formations can deliver predictable steam without excessive engineering.
But, ironically, it is the fracking boom, which has helped the oil & gas industries get access to new sources of carbon-producing energy, which may help us tap geothermal power in more places. As fracking and oil & gas exploration has led to a revolution in our ability to precisely drill deep underground and push & pull fluids, it also presents the ability for us to tap more geothermal power than ever before. This has led to the rise of enhanced geothermal, the process by which we inject water deep underground to heat, and leverage the steam produced to generate electricity. Studies suggest the resource is particularly rich and accessible in the Southwest of the United States (see map below) and could be an extra tool in our portfolio to green energy consumption.
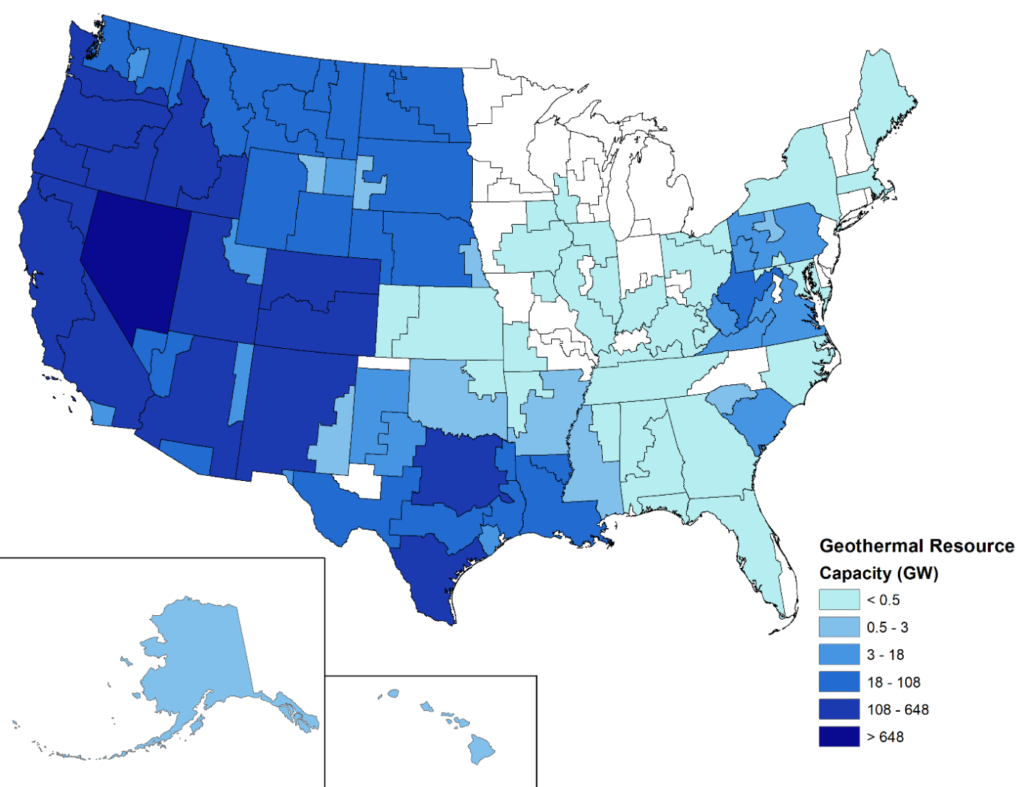
While there is a great deal of uncertainty around how much this will cost and just what it will take (not to mention the seismic risks that have plagued some fracking efforts), the hunger for more data center capacity and the desire to power this with clean electricity has helped startups like Fervo Energy and Sage Geosystems fund projects to explore.
On 17 October, Fervo Energy, a start-up based in Houston, Texas, got a major boost as the US government gave the green light to the expansion of a geothermal plant Fervo is building in Beaver County, Utah. The project could eventually generate as much as 2,000 megawatts — a capacity comparable with that of two large nuclear reactors. Although getting to that point could take a while, the plant already has 400 MW of capacity in the pipeline, and will be ready to provide around-the-clock power to Google’s energy-hungry data centres, and other customers, by 2028. In August, another start-up, Sage Geosystems, announced a partnership with Facebook’s parent company Meta to deliver up to 150 MW of geothermal power to Meta’s data centres by 2027.
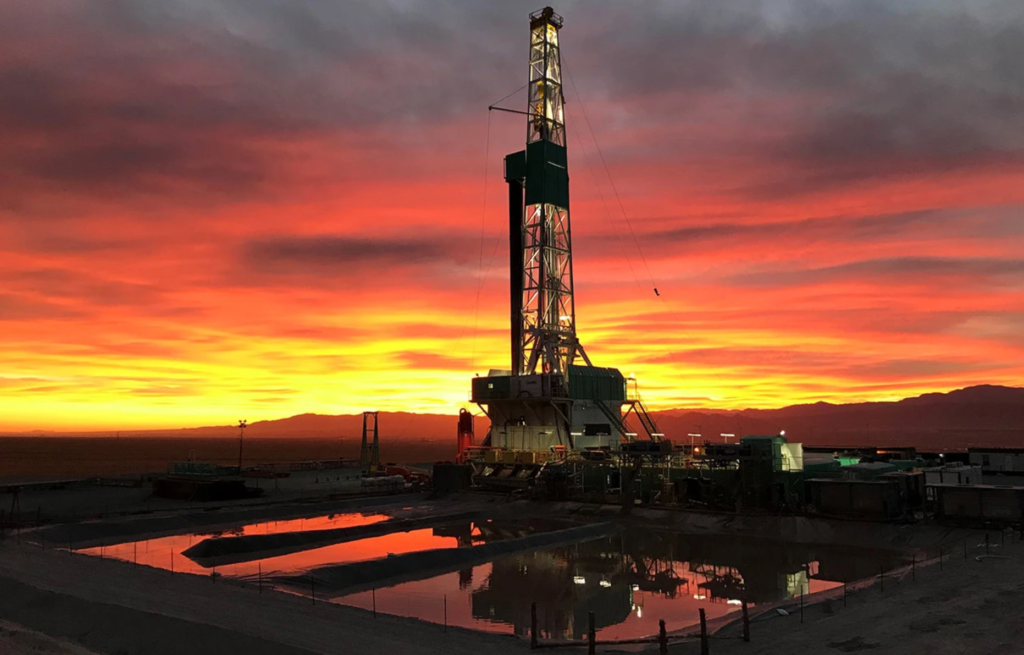
Geothermal power is vying to be a major player in the world’s clean-energy future
David Castelvecchi | Nature News